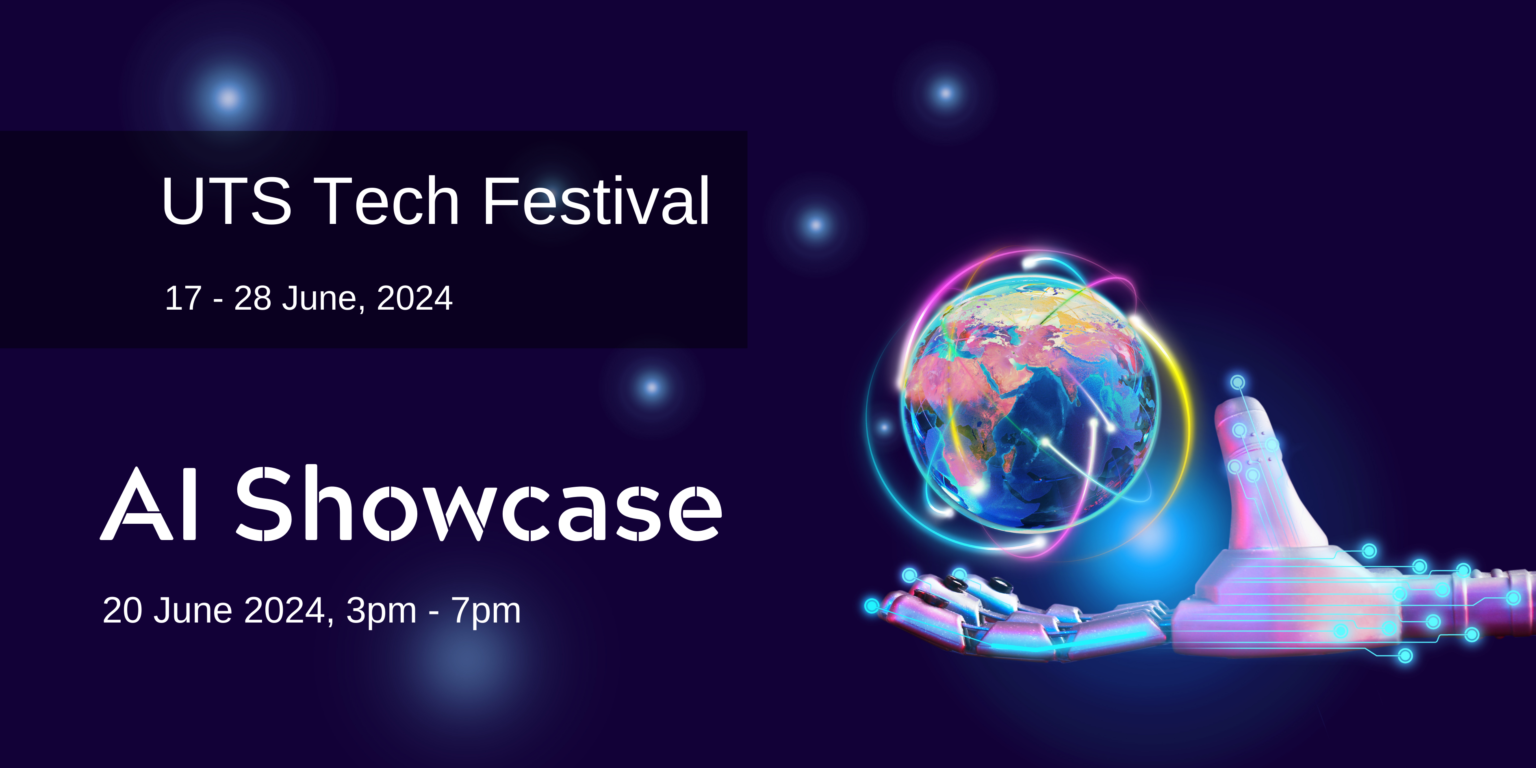
About
UTS Tech Festival (17 – 28 June, 2024) is a two weeks festival of events, showcases, hackathons, workshops, masterclasses, seminars, competitions, industry-student engagement and much more! Our goal is to bring together students, academics and industry to foster learning, inspiration, share ideas and promote innovation.
Come along to the UTS AI Showcase which is part of the UTS Tech Festival 2024, to discover Artificial Intelligence, Deep Learning, Reinforcement Learning, Computer Vision and NLP projects!
This the third iteration of the UTS AI Showcase that showcases Artificial Intelligence (AI) and Deep Learning projects developed by undergraduate, postgraduate, and higher degree research (HDR) students from the Faculty of Engineering and IT (FEIT).
Venue:
UTS Faculty of Engineering and Information Technology
Broadway, Ultimo, NSW 2007, Australia
Date and Time:
Thursday, June 20, 2024, 3.00pm – 7.00pm
Showcase Winners
Showcase Winners
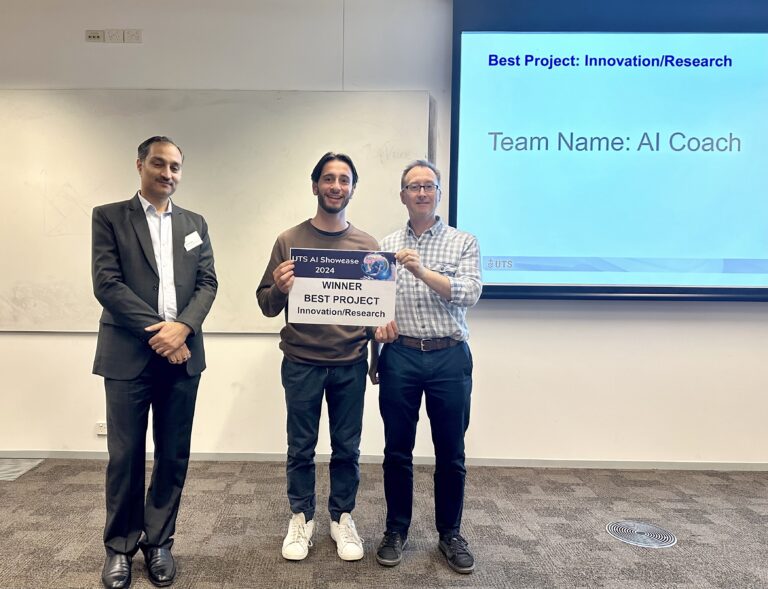
Best Project:
Innovation/Research
Team: AI Coach
Team Members:
Christopher Armenio
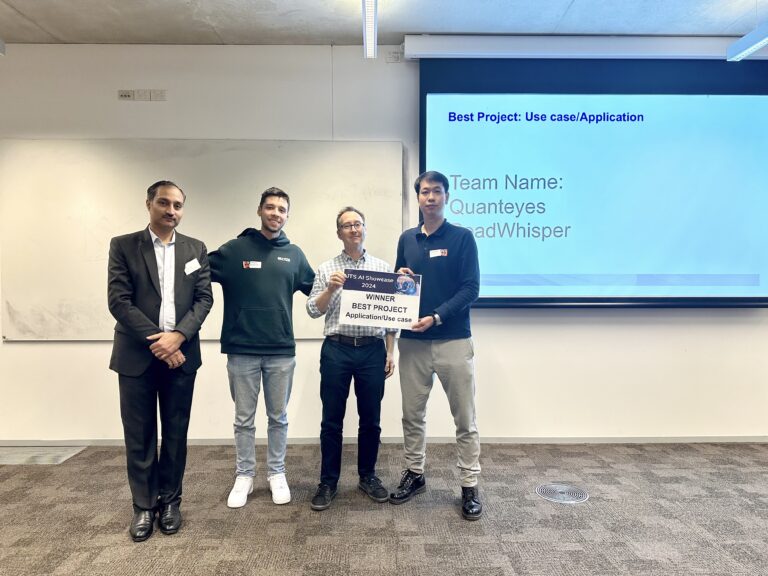
Best Project: Usecase/Application
Team: Quanteyes
Team Members:
Jordan Stojcevski
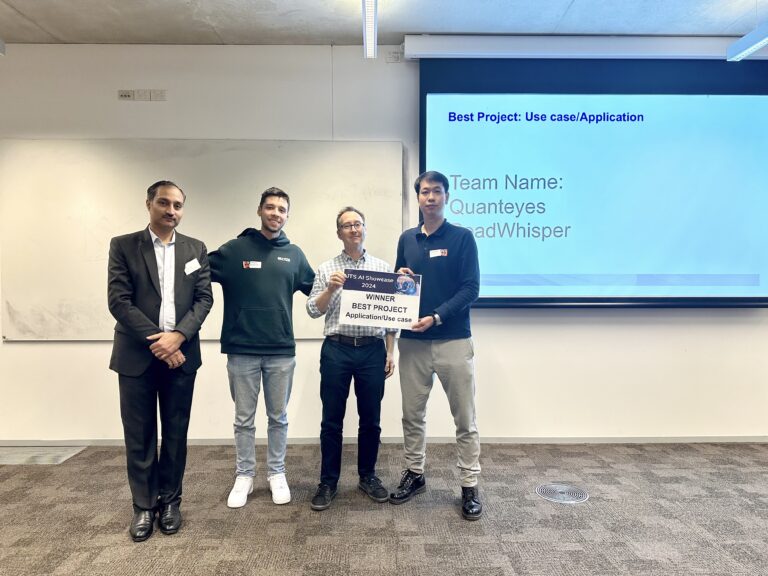
Best Project: Usecase/Application
Team: RoadWhisper
Team Members:
Trong Nghia Tran
Binh An Thai
Rozhin Vosoughi
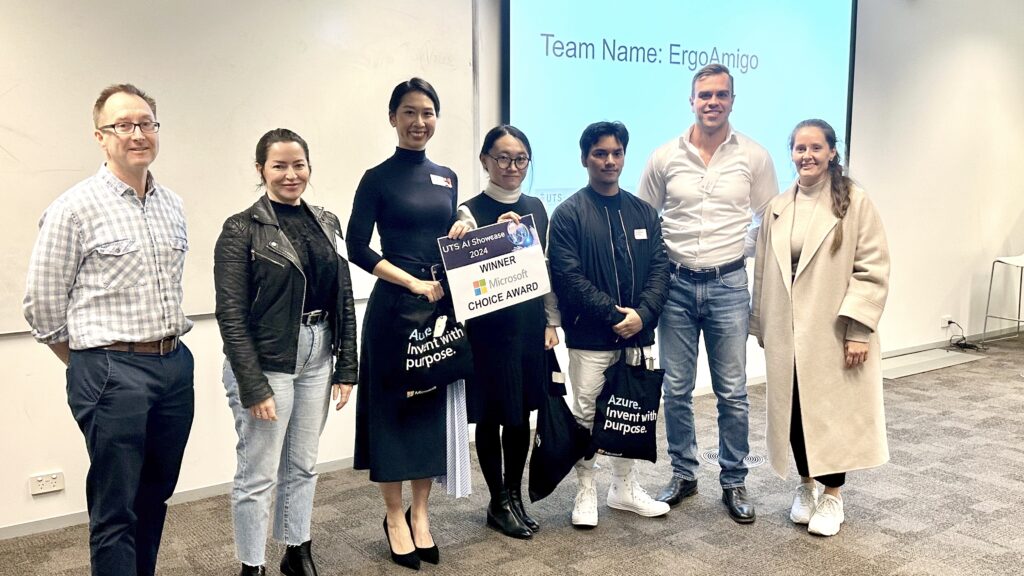
Microsoft Industry Choice Award
Team: ErgoAmigo
Team Members:
Yen Chan
Yan(Amy) Yang
Leonardo Rodriguez Magana
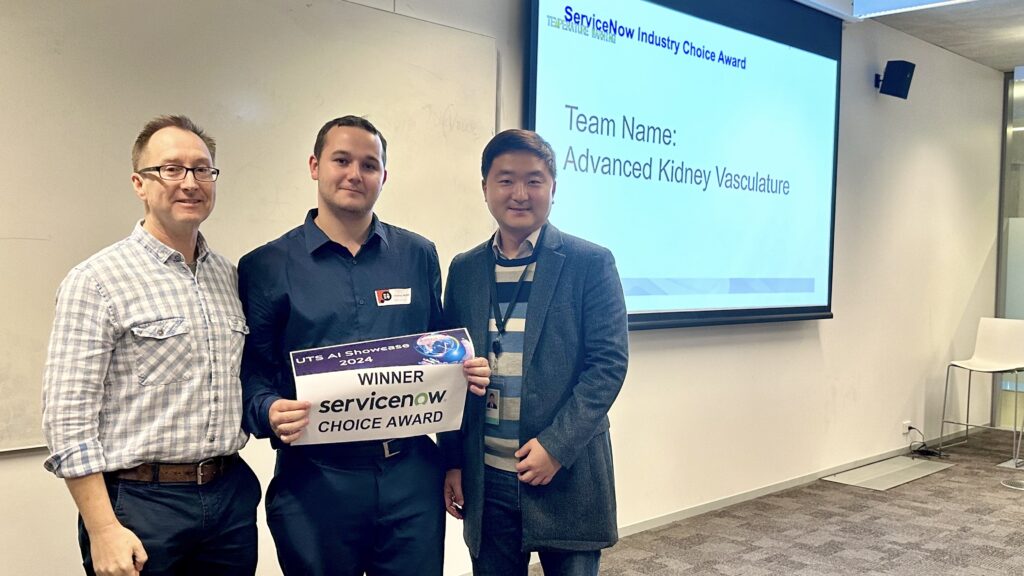
ServiceNow Industry Choice Award
Team: Advanced Kidney Vasculature
Team Members:
Jonathan Morel
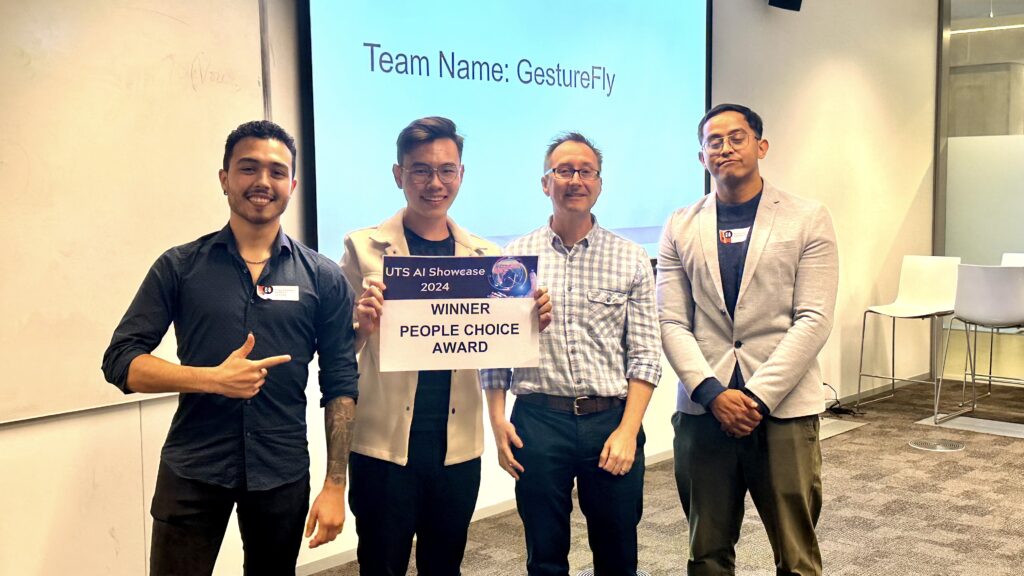
People Choice Award
Team: GestureFly
Team Members:
Diego Alejandro Ramirez Vargas,
Fendy Lomanjaya
Shudarshan Kongkham
Participating Teams
Teams from Deep Learning and CNN subject
Gesture Fly
CNN based Gesture Recognition for Tello Drone Command Execution and Face Recognition for Authentication. The actions trained for controlling the drone are based on the navigation functions of the Tello drones. The novelty of the project lies in the authentication of the person, thereby securing the control of their drone itself.
Room : CB11.04.205
Pod : 1
RoadWhisper
Driving is common, but staying fully aware is challenging, especially when tired or in unfamiliar areas. This can lead to distracted driving and missed signs or animal collisions. Our project uses advanced computer vision and deep learning to assist drivers, enhancing situational awareness, improving safety, reducing fines, and protecting wildlife.
Room : CB11.04.205
Pod : 2
EmotionAI
Emotion is crucial for building social connections and a sense of belonging. People with ASD often struggle with understanding emotions, affecting social interactions. EmotionAI uses computer vision for instant emotion recognition via a camera, enhancing spontaneous social connections in daily life.
Room : CB11.04.400
Pod : 1
Lens Linkup
Our innovative Lens Linkup project offers a virtual try-on system for contact lenses, filling the gap in pre-purchase trials. Using a CNN via a web app, we digitally superimpose lenses onto users' eyes in videos and images. Our approach maximizes reach and informs purchasing decisions with breakthrough accuracy and overlay fidelity.
Room : CB11.04.400
Pod : 2
Herd Watch
Livestock management is crucial in agriculture, needing efficient methods for counting and monitoring behavior. Traditional manual approaches are time-consuming, costly, and error-prone. This project aims to develop a system using computer vision algorithms for livestock counting and behavior detection.
Room : CB11.04.400
Pod : 3
FruitTale
"FruitTale" is an innovative web platform for toddlers, teaching them about fruits through interactive experiences. Using object detection via webcam, it identifies fruits shown by children and offers educational content, including fun facts and nutrition info. The platform employs a CNN trained on diverse fruit datasets for accurate, unbiased recognition.
Room : CB11.04.400
Pod : 4
LaTeX Converter
In the digital age, converting handwritten math equations into LaTeX is crucial but challenging, especially for students. This was evident during the COVID-19 lockdown, requiring students to digitize their solutions. We propose a CNN to convert handwritten equations into LaTeX, ensuring consistent, readable output and enhancing the educational experience.
Room : CB11.04.400
Pod : 6
Multi-Person Video2Transcript
The project aimed to develop an AI system to automatically generate transcripts from multi-person videos like YouTube or professional broadcasts, enhancing searchability and keyword generation. Using a combination of CNN, HOG and RNN for speech detection, the system extracts spoken text and produces annotated videos with transcripts.
Room : CB11.04.400
Pod : 7
ErgoAmigo
ErgoAmigo, a virtual ergonomic assistant, optimizes workstations for hybrid and remote workers globally. Offering real-time assessments and guidance on adjustments, it emphasizes sitting posture and screen-to-face distance. The app utilizes computer vision models and mathematical methods for automated ergonomic assessment, with potential for broader applications in ergonomic evaluations.
Room : CB11.04.400
Pod : 8
RaceTec
Traditional race bib identification relies on costly RFID technology and specialized readers, making upgrades challenging. We've developed a cost-effective solution using computer vision to read bib numbers. This approach is accessible and can run on most available computers, complementing traditional detection methods effectively.
Room : CB11.04.400
Pod : 9
FocusAI
FocusAI employs a CNN model to autonomously identify individuals in medium-density crowds, inspired by smartphone portrait modes. It overcomes traditional imaging limitations by automating clarity enhancement and focusing on individuals. The solution it offers significant applications in photography, security, and crowd management, advancing computer vision.
Room : CB11.04.400
Pod : 10
GradeGenius AI
GradeGenius AI provides hand-held, automated, multiple choice marking, utilising the latest Object Detection technologies. Our offline solution allows teachers and academics to promptly mark multiple choice exam papers, allowing faster returning of marks to students. Our interface allows for easy comparisons, and fast analysis, ensuring peace of mind for the teachers and students alike.
Room : CB11.04.400
Pod : 11
Disaster Assist Drone
The Disaster Assist Drone (D.A.D.) project uses a U-Net CNN for real-time road condition monitoring during disasters. Mounted on drones, it identifies traversable paths, aiding rescue teams. The user-friendly GUI, developed with Tkinter, enables easy image import and analysis. D.A.D. extends to urban planning, infrastructure, and logistics, revolutionizing disaster management.
Room : CB11.04.400
Pod : 12
Abandoned Luggage detection
Our goal is to improve security by detecting abandoned luggage, aiding in tracking lost items or identifying potential threats. The primary use case is in airports or crowded areas. We developed a PC application connected to CCTV systems for real-time detection, utilizing the Faster-RCNN algorithm.
Room : CB11.04.400
Pod : 13
Tidetip
Our solution aims to automate rip current detection, replacing manual methods. By connecting to beach cameras, our algorithm detects rip currents in real time, ensuring precision and safety. This innovation allows beach authorities to safeguard visitors and promote responsible beach trips effortlessly.
Room : CB11.04.400
Pod : 14
LeafLens
LeafLens is a mobile application that uses AI-driven image segmentation to identify diseased plant areas. It serves agricultural professionals and gardeners, enabling early disease detection, reducing crop loss, and enhancing productivity through timely interventions, all accessible directly via smartphones.
Room : CB11.04.400
Pod : 15
RealTime Traffic Speed Detection
Our real-world speed detection project employs color-coded boxes to identify speeding and moving vehicles, providing real-time speed display. By analyzing vehicle movement, it accurately detects speed violations, enhancing road safety and enforcement. Provides visual indication of speeding vehicles, make is easier for realtime surveillance and monitoring of road safety.
Room : CB11.04.300
Pod : 1
AI-Coach
Our AI sports analyzer program combines LSTM networks and Google MediaPipe's pose detection to offer precise biomechanical feedback. It captures and analyzes an athlete's running form on smartphones, extracting key body landmarks for a skeletal model. By comparing this model against ideal parameters, it provides real-time corrective insights for posture and stride optimization, democratizing advanced sports analytics for all athletes.
Room : CB11.04.300
Pod : 2
Pokebrokers
In the modern trading card community, Pokemon card collection has surged. Each card's value depends on its rarity, popularity, and competitive viability, causing prices to fluctuate. Bargaining is common, but time-consuming. Our project creates a platform to scan cards, displaying real-time prices, streamlining trading and purchasing decisions, and reducing tediousness.
Room : CB11.04.300
Pod : 3
Art Restoration Using CNN
Artworks often suffer damage from environmental factors, accidents, or vandalism, risking their integrity. Traditional restoration is slow and costly. This project uses deep learning to automate image inpainting, restoring damaged areas seamlessly. Our CNN model aims to preserve cultural heritage, benefiting galleries and museums by providing efficient, scalable restoration solutions.
Room : CB11.04.300
Pod : 4
Where am I at UTS
"Where am I at UTS" revolutionizes indoor navigation at the University of Technology Sydney. Using AI and neural networks, the app utilizes the mobile camera to provide indoor positioning and navigation inside UTS to allow students, staff, and visitors to easily navigate the campus with confidence and ease.
Room : CB11.04.301
Pod : 7
Quanteyes
Quanteyes has built a motorcycle logo detection system that is used within a digital asset management system to tag assets as they are uploaded to cloud storage. This makes it easy for social media managers to filter, manage and organise their content from around the world.
Room : CB11.04.301
Pod : 8
Teams from Computer Science Studio subject
AI Content Detection
I have created a Text-Convolutional Neural Network to detect AI written essays and used contrastive feature extraction to detect AI generated images. These are both in order to address the challenge of dealing with dishonest work in the new AI era. Here is my short presentation and demo: https://youtu.be/06ZQYE8AGDA
Room : CB11.04.300
Pod : 5
Advanced Kidney Vasculature
Our project, Advanced Kidney Vasculature Segmentation Using Deep Learning, maps kidney vascular structures with a U-Net neural network using 2.5D CT images. This method improves accuracy over traditional and outdated automated segmentation. Advanced data augmentation ensures adaptability, achieving a Dice coefficient of 0.81, enhancing diagnostics, disease detection, and surgical planning.
Room : CB11.04.301
Pod : 1
Using AI To Detect Cyberattacks In Real-Time
Cyberattacks are a current reoccurring issue. Our group solves this by training an AI model to detect cyberattacks in real-time, and this is done by sniffing and analysing the type of data packets that is entering into your computer. Our program will alert you to take safeguarding measures if an attack is observed.
Room : CB11.04.301
Pod : 2
Teams from Artificial intelligence Studio subject
Automated Safety Equipment Detection System (ASEDS)
ASEDS is AI system to detect safety gear on construction sites, including helmets, vests, and 23 other items. This system helps to minimize hazards, improves regulatory adherence, and reduces insurance costs. Experience a smarter, safer work environment with ASEDS, the future of site safety monitoring.
Room : CB11.04.301
Pod : 3
BrainScan
Early detection of brain tumors is crucial for effective treatment, but current methods are slow and error-prone. BrainScan uses AI to analyze MRI scans, providing instant, accurate diagnostics via a mobile app. This improves access in underserved areas, integrates with medical workflows, and enhances diagnostic precision and patient outcomes.
Room : CB11.04.301
Pod : 5
Teams from Natural Language Processing subject
Semi-automated Recognition of Prior Learning
Our project revolutionizes the Recognition of Prior Learning (RPL) process with Natural Language Processing. Using Sentence Transformer Embeddings, we've automated RPL, allowing efficient mapping of past experiences to new opportunities. The application offers tailored recommendations for students and universities, enhancing flexibility and adaptability in education. Join us in shaping education's future.
Room : CB11.04.301
Pod : 6
Teams from Reinforcement Learning subject
Racing AI
RacingAI is an innovative AI system designed to enhance autonomous driving through Reinforcement Learning. Utilizing the Donkey Car Simulator and the JetRacer AI Kit, this system improves adaptive decision-making and continuous learning in self-driving cars. Experience the new phase of autonomous driving with RacingAI, revolutionizing vehicle intelligence and performance.
Room : CB11.04.301
Pod : 4
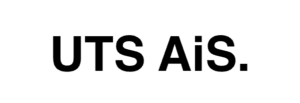
UTS Artificial Intelligence(AI) Society
Introducing the UTS AiS
We are a student-led Artificial Intelligence (AI) society. Our mission is to foster relationships across the university, allowing teams of students to engage with academics and industry professionals about how AI is affecting their current disciplines and future work. We have many planned activities designed to inspire growth and learning, including workshops, tutorials, networking events, talks, and more. We welcome everyone to join, whether you are a STEM student or studying law, business, or any other field. Our aim is to teach, help, and inspire everyone to be innovative and push the frontiers of AI further.
Room : CB11.04.Foyer
JOIN US
Meet Our Judges
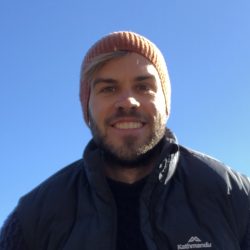
Keppell Smith
Microsoft
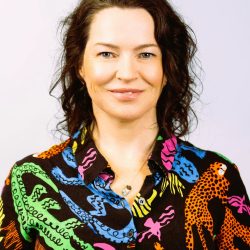
Sinéad Fitzgerald
Microsoft
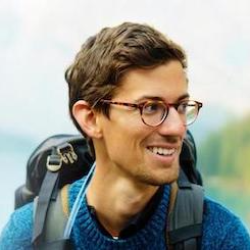
Guillaume Jounel
AI Lead, DroneShield
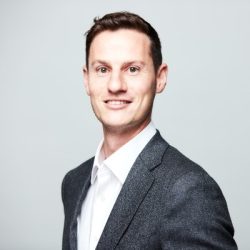
Alex Hoser
ServiceNow
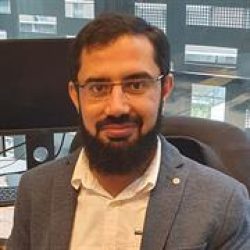
Dr. Muhammad Saqib
CSIRO/Data61

A/Prof. Hai Yan Lu
University of Technology Sydney
Meet Our Industry Speakers
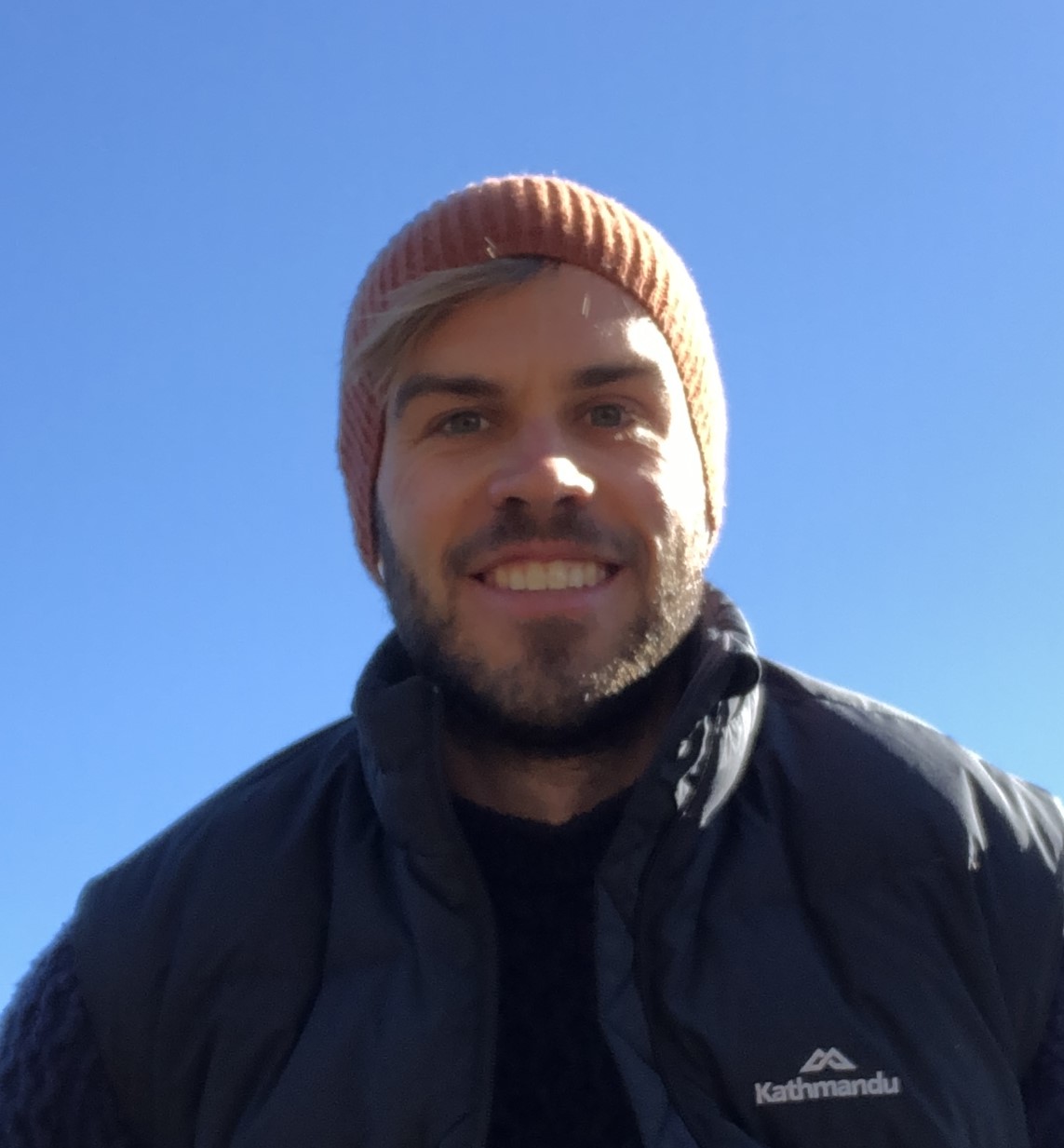
Keppell Smith
Keppell is an experienced startup professional with a rich experience as an exited fintech founder and ANZ Startups Director at Microsoft. Currently leading a portfolio of AU & NZ’s 40 highest-potential startups, he also manages Microsoft Aisa’s AI taskforce, playing a crucial role in executing the global AI strategy. Outside of work he enjoys hiking, swimming and reading (mostly history & economics) as well as keeping active in the angel investor community
Sinéad Fitzgerald
Sinéad Fitzgerald leads Microsoft ANZ's Digital Health and EduTech ISV Partnerships for the region. With a strong background in strategic partnerships and technology, she connects Microsoft with the innovative startup community across Australia and New Zealand. Sinéad is an accomplished presenter and inspiring social leader, deeply fascinated by how technology and human connection can enrich lives.
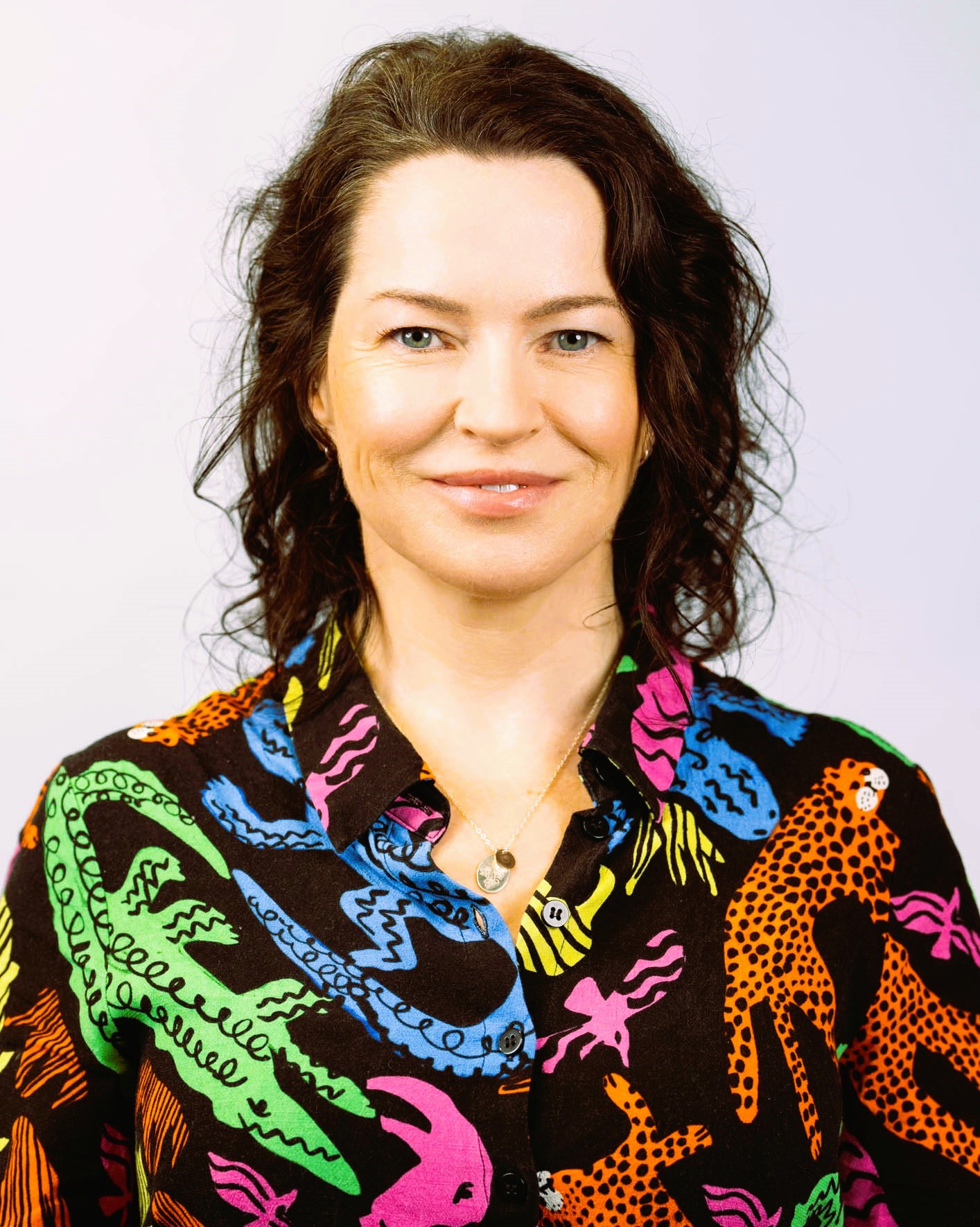
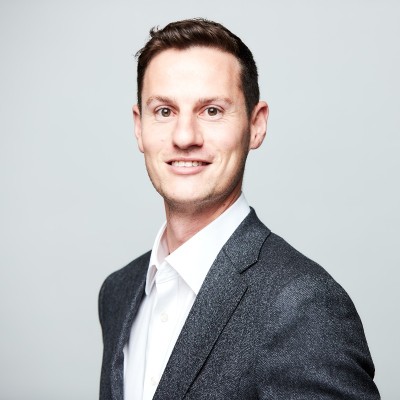
Alex Hoser
Alex is the Senior Sales Manager for State Government and Education at ServiceNow.
Organizing Committee
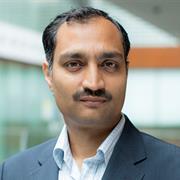
A/Prof. Nabin Sharma
Organizing Chair and Lead
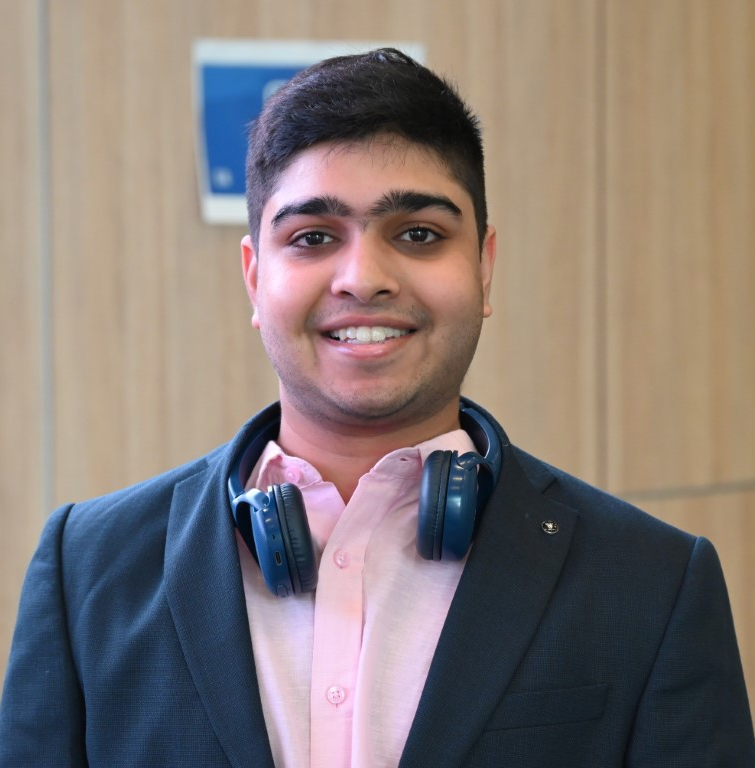
Gitarth R Vaishnav
Casual Academic
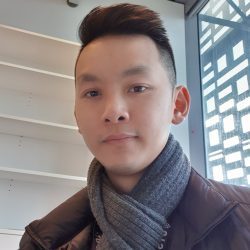
Jason Do
Coodinator, Student Engagement

Sarah Rodriguez
Student Engagement Officer
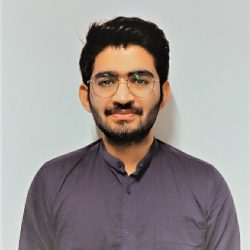
Vishesh Sompura
Event Assistant
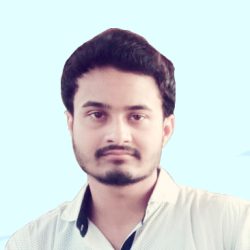
Arnab Haldar
Photography Assistant
Supported By
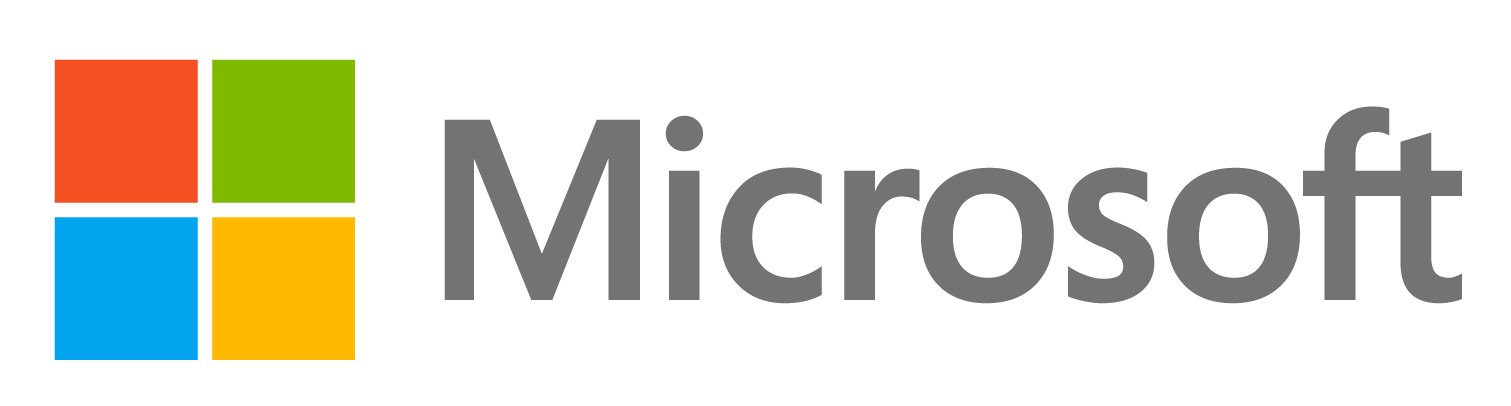
